Which Table Of Values Represents The Residual Plot
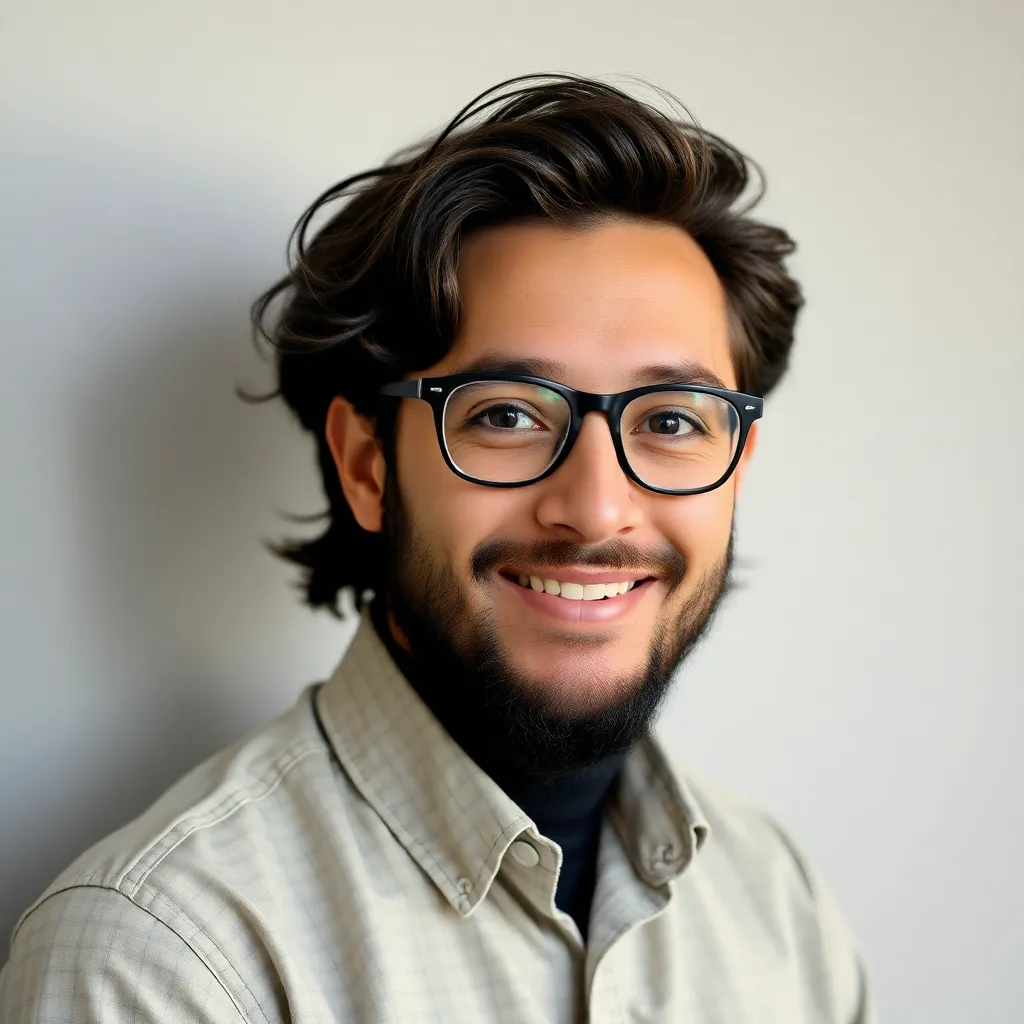
Breaking News Today
Apr 08, 2025 · 6 min read

Table of Contents
Which Table of Values Represents the Residual Plot? Understanding Residuals in Regression Analysis
Understanding residual plots is crucial for assessing the validity and reliability of a regression model. A regression model aims to describe the relationship between a dependent variable and one or more independent variables. However, the model is rarely perfect; there's always some unexplained variation. This unexplained variation is captured in the residuals. Therefore, knowing which table of values represents a residual plot is paramount to evaluating the model's fitness. This comprehensive guide will delve into the concept of residuals, explain how to identify them in a table, and discuss the importance of interpreting residual plots for accurate model assessment.
What are Residuals?
Residuals, also known as errors, represent the difference between the observed value of the dependent variable and the value predicted by the regression model. In simpler terms, it's the discrepancy between what actually happened and what the model predicted would happen. A smaller residual indicates a better fit, while a larger residual suggests a poorer fit. Mathematically, the residual (eᵢ) for the i-th observation is calculated as:
eᵢ = yᵢ - ŷᵢ
Where:
- yᵢ is the observed value of the dependent variable for the i-th observation.
- ŷᵢ is the predicted value of the dependent variable for the i-th observation, as determined by the regression model.
Identifying Residuals in a Table of Values
A table representing a residual plot will typically include at least these three columns:
-
Independent Variable (x): This column lists the values of the independent variable used in the regression analysis. This is the variable that's believed to influence the dependent variable.
-
Dependent Variable (y): This column shows the observed values of the dependent variable. These are the actual data points collected.
-
Residuals (e): This crucial column contains the calculated residuals – the difference between the observed (y) and predicted (ŷ) values. This is the core element that differentiates a simple data table from a table representing a residual plot. A properly constructed residual table will explicitly show this residual column.
Example:
Let's consider a simple linear regression model where we're trying to predict house prices (y) based on their size (x). A hypothetical table representing a residual plot might look like this:
House Size (x) (sq ft) | House Price (y) ($) | Predicted Price (ŷ) ($) | Residual (e) ($) |
---|---|---|---|
1500 | 250000 | 240000 | 10000 |
1800 | 300000 | 280000 | 20000 |
2000 | 320000 | 300000 | 20000 |
2200 | 350000 | 320000 | 30000 |
2500 | 400000 | 370000 | 30000 |
In this example, the "Residual (e)" column is what identifies this table as one that can be used to create a residual plot. Note that the "Predicted Price (ŷ)" column isn't strictly necessary for the residual plot itself but is helpful in understanding the calculation of residuals.
Interpreting Residual Plots: Visualizing the Model's Fit
While a table of residuals provides the numerical data, a residual plot offers a visual representation that's far more insightful. A residual plot graphs the residuals (e) against the predicted values (ŷ) or the independent variable (x). The interpretation of the plot is key to evaluating the model's assumptions and its overall goodness-of-fit.
Key Features to Look For in a Residual Plot:
-
Random Scatter: Ideally, the residuals should be randomly scattered around zero. No discernible pattern should emerge. If a pattern exists (e.g., a curve, a clear trend), it suggests that the model's assumptions are violated.
-
Constant Variance (Homoscedasticity): The spread of the residuals should be roughly constant across the range of predicted values or the independent variable. If the spread increases or decreases systematically (heteroscedasticity), it indicates that the model's variance is not constant, a violation of a key assumption of linear regression.
-
Outliers: Individual points that are far away from the rest of the points (large residuals) might represent outliers in the data. These outliers need to be carefully examined; they might be data entry errors or genuinely influential data points that require further investigation.
-
Non-Linearity: If the residual plot shows a curved pattern, it suggests that the relationship between the dependent and independent variables isn't linear. A non-linear model might be more appropriate.
-
Independence of Errors: The residuals should be independent of each other. A pattern suggesting autocorrelation (correlation between consecutive residuals) might indicate a problem with the model's assumptions, especially in time series data.
How to Identify if a Table Represents a Residual Plot Summary
To determine if a table represents a residual plot, look for the following:
- Presence of Residuals: The most crucial element is the explicit presence of a column labeled "Residuals," "Errors," or a similar term, containing the calculated differences between observed and predicted values.
- Contextual Information: The table should be presented within the context of a regression analysis. It should be accompanied by information on the regression model used (linear, polynomial, etc.), the dependent and independent variables, and the statistical metrics (R-squared, adjusted R-squared, etc.).
- Statistical Significance: A robust analysis will include information about the significance of the model and its coefficients. This information helps in judging the overall reliability of the residual analysis.
Advanced Considerations and Extensions
Leverage and Influence: While not always explicitly in the table itself, leverage and influence are closely related concepts. High leverage points are those with extreme values of the independent variables. Influential points are those that significantly impact the regression model. Identifying these points requires further statistical analysis beyond a simple residual table, but it is crucial for understanding the model's robustness.
Transformations: If the residual plot shows violations of assumptions (e.g., non-constant variance, non-linearity), transformations of the variables might be necessary to improve the model's fit. Common transformations include logarithmic, square root, and reciprocal transformations.
Multiple Regression: The principles described above extend to multiple regression models (models with more than one independent variable). Interpreting residual plots in multiple regression can be more complex due to the higher dimensionality, often requiring graphical techniques to assess the assumptions.
Conclusion: The Importance of Understanding Residual Plots
A table of values representing a residual plot is a cornerstone of assessing the validity and reliability of a regression model. While the numerical values in the table provide the raw data, the visual representation of the residual plot offers critical insights into the model's fit and its adherence to underlying assumptions. By carefully examining the patterns (or lack thereof) in the residual plot, you can determine whether the model accurately represents the data or needs refinement. Understanding residuals is not just a statistical exercise; it's a crucial aspect of ensuring the conclusions drawn from a regression analysis are accurate, reliable, and trustworthy. Paying close attention to the residual table and its graphical representation is paramount to building robust and effective regression models. Remember to always consider the context in which the residual table is presented to ensure a comprehensive understanding. The inclusion of relevant statistical metrics and discussions about model fit strengthens the analysis significantly.
Latest Posts
Latest Posts
-
What Is The Best Most Accurate Description For Agonal Respirations
Apr 08, 2025
-
Physical And Chemical Properties And Changes Answer Key
Apr 08, 2025
-
Which Of The Following Might Trigger Erythropoiesis
Apr 08, 2025
-
Strength Training Makes Ligaments Tendons And Cartilage Stronger
Apr 08, 2025
-
What Is The Main Goal Of The Presidents Challenge Program
Apr 08, 2025
Related Post
Thank you for visiting our website which covers about Which Table Of Values Represents The Residual Plot . We hope the information provided has been useful to you. Feel free to contact us if you have any questions or need further assistance. See you next time and don't miss to bookmark.