In-sample And Out-of-sample Criteria Are Based On The Forecast '
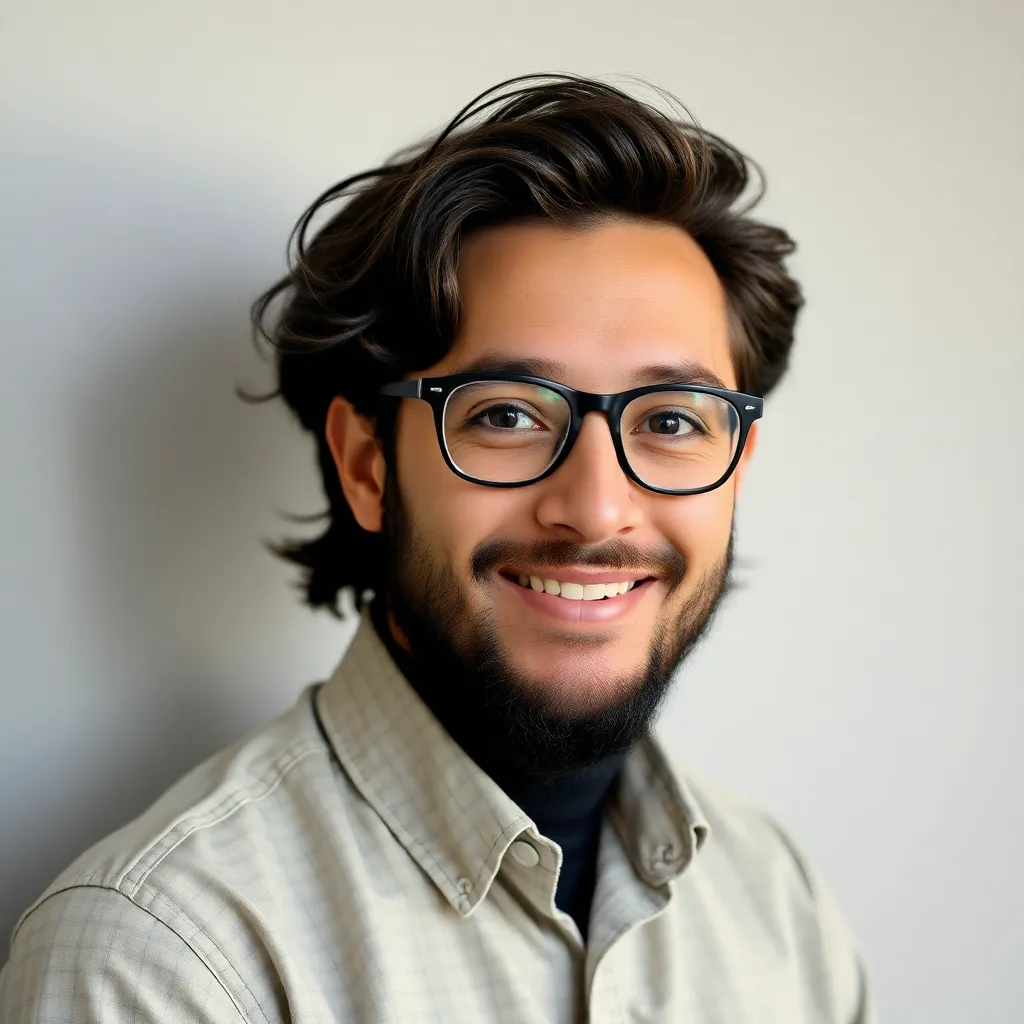
Breaking News Today
Apr 01, 2025 · 6 min read
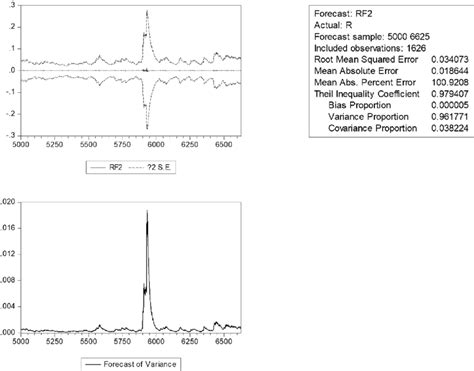
Table of Contents
In-Sample and Out-of-Sample Criteria: Evaluating Forecast Accuracy
Forecasting is a crucial aspect of numerous fields, from finance and economics to meteorology and supply chain management. The accuracy of a forecast directly impacts decision-making, and therefore, rigorous evaluation methods are paramount. This article delves into the core concepts of in-sample and out-of-sample criteria, explaining their significance in assessing forecast performance and guiding the selection of appropriate forecasting models. We'll explore various evaluation metrics and discuss the practical implications of each approach.
Understanding In-Sample and Out-of-Sample Data
Before diving into the criteria, it's crucial to understand the distinction between in-sample and out-of-sample data. This distinction forms the bedrock of evaluating forecast accuracy and preventing overfitting.
In-Sample Data: The Training Ground
In-sample data refers to the historical data used to train or estimate the parameters of a forecasting model. This is the dataset the model "learns" from. The model is adjusted and optimized based on this data to minimize prediction errors within the sample.
Think of it as the model's practice session. It's where the model learns the patterns and relationships within the data to make future predictions.
Out-of-Sample Data: The Real Test
Out-of-sample data, on the other hand, comprises data not used during the model's estimation process. It represents future or unseen data points. Evaluating the model's performance on out-of-sample data provides a more realistic assessment of its predictive capabilities. This is because it measures how well the model generalizes to new, unseen data, rather than simply memorizing the training data.
This is the model's final exam. It shows how well the model performs on data it hasn't seen before, which is the true measure of its forecasting ability.
In-Sample Criteria: Assessing Model Fit
In-sample criteria assess how well the model fits the historical data used for its estimation. While seemingly straightforward, it's essential to interpret in-sample criteria cautiously. A model with excellent in-sample performance might still exhibit poor out-of-sample performance due to overfitting. Overfitting occurs when the model learns the noise or random fluctuations in the training data, rather than the underlying patterns. This leads to high accuracy within the sample but low accuracy in predicting future values.
Common In-Sample Criteria:
-
Root Mean Squared Error (RMSE): This measures the average difference between the predicted and actual values, taking into account the magnitude of the errors. A lower RMSE indicates better in-sample fit.
-
Mean Absolute Error (MAE): Similar to RMSE, MAE measures the average absolute difference between predicted and actual values. It's less sensitive to outliers than RMSE.
-
Mean Absolute Percentage Error (MAPE): This expresses the average absolute percentage difference between predicted and actual values. It provides a relative measure of accuracy, making it easier to compare across different datasets.
-
R-squared: This statistic measures the proportion of variance in the dependent variable explained by the model. A higher R-squared suggests a better in-sample fit, but it shouldn't be the sole criterion for model selection.
Limitations of In-Sample Criteria:
- Overfitting: High in-sample accuracy doesn't guarantee good out-of-sample accuracy. A model can overfit the training data and perform poorly on new data.
- No indication of future performance: In-sample metrics only reflect the model's ability to fit the past data. They don't necessarily predict future performance.
- Data dependence: The performance of in-sample metrics can be highly dependent on the characteristics of the training data.
Out-of-Sample Criteria: Evaluating Predictive Power
Out-of-sample criteria are crucial for assessing a model's true predictive power. They evaluate the model's ability to accurately predict future values based on its performance on unseen data. This provides a far more realistic measure of the model's efficacy and avoids the pitfalls of overfitting.
Common Out-of-Sample Criteria:
The same metrics used for in-sample evaluation (RMSE, MAE, MAPE, R-squared) can also be applied to out-of-sample data. However, the interpretation changes significantly. For example, a low out-of-sample RMSE indicates a model that accurately predicts future values.
Techniques for Out-of-Sample Evaluation:
-
Holdout Method: A portion of the data is withheld from the model training and used for testing. This is a simple but effective technique.
-
Time Series Cross-Validation: This method is particularly useful for time-series data. The data is divided into multiple segments, with each segment used as a test set after training the model on the preceding segments. This allows for evaluating the model's performance over time.
-
Rolling Window: Similar to time series cross-validation, but the window size is fixed, and it "rolls" through the data, creating multiple training and testing sets.
Importance of Out-of-Sample Evaluation:
- Predictive Power: It provides a direct measure of the model's ability to predict future values.
- Generalization Ability: It assesses the model's ability to generalize to new, unseen data.
- Overfitting Detection: Significant discrepancies between in-sample and out-of-sample performance indicate potential overfitting.
- Model Selection: Out-of-sample performance is crucial for selecting the best model among competing candidates.
Choosing the Right Forecasting Model: A Balanced Approach
Selecting the "best" forecasting model involves a careful consideration of both in-sample and out-of-sample criteria. A model with superior in-sample performance isn't necessarily the best choice if it suffers from overfitting and performs poorly out-of-sample.
The ideal model balances good in-sample fit with strong out-of-sample predictive power. This often involves exploring various model types and using techniques like cross-validation to ensure robustness. It's not always about achieving the absolute lowest error; it's about finding the model that provides the most reliable and actionable predictions for the specific application.
Beyond the Metrics: Practical Considerations
While the metrics discussed above are critical, it’s important to consider other practical aspects when evaluating forecasts:
-
Understanding the Data: The quality and characteristics of the data significantly impact forecast accuracy. Outliers, missing values, and data transformations should be carefully considered.
-
Business Context: The chosen metrics and model should align with the specific business needs and goals. A model that minimizes RMSE might not be the most suitable if the business prioritizes minimizing the risk of large errors.
-
Interpretability: In some applications, the interpretability of the model is just as important as its accuracy. A complex model with high accuracy might be less useful if its predictions are difficult to understand and explain.
-
Computational Cost: The computational cost of different models should also be considered, especially when dealing with large datasets or real-time forecasting requirements.
Conclusion: A Holistic Approach to Forecast Evaluation
In-sample and out-of-sample criteria are essential tools for evaluating the performance of forecasting models. While in-sample criteria provide insights into how well a model fits the historical data, out-of-sample criteria offer a more realistic assessment of its predictive power and ability to generalize to new data. A balanced approach, considering both criteria along with practical considerations, is crucial for selecting and implementing effective forecasting models that meet the specific needs of a given application. By understanding and applying these concepts effectively, we can enhance the accuracy and reliability of our forecasts, leading to better decision-making and improved outcomes.
Latest Posts
Latest Posts
-
Which Word Best Describes Early American Foreign Policy
Apr 02, 2025
-
You Become An Assigned Risk Driver When
Apr 02, 2025
-
Immigrants From Different Ethnic Groups Have Different
Apr 02, 2025
-
You Should In Order To Document Data Properly
Apr 02, 2025
-
Which Statement Accurately Describes Type 2 Diabetes
Apr 02, 2025
Related Post
Thank you for visiting our website which covers about In-sample And Out-of-sample Criteria Are Based On The Forecast ' . We hope the information provided has been useful to you. Feel free to contact us if you have any questions or need further assistance. See you next time and don't miss to bookmark.