Inferences Based On Voluntary Response Samples Are Generally Not Reliable.
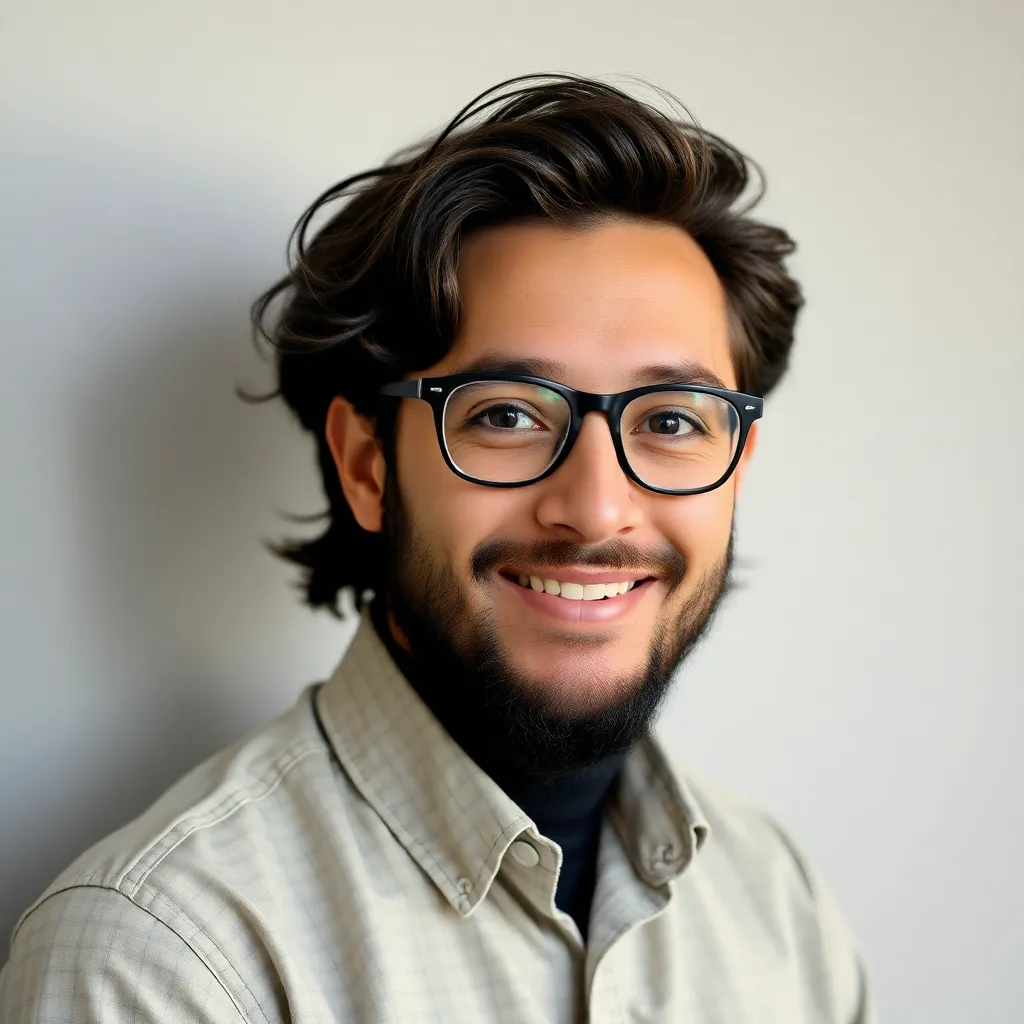
Breaking News Today
Apr 03, 2025 · 6 min read

Table of Contents
Inferences Based on Voluntary Response Samples Are Generally Not Reliable
Voluntary response samples, also known as self-selected samples, are a common form of data collection where individuals choose themselves to participate in a survey or study. While seemingly convenient and cost-effective, inferences based on these samples are generally unreliable and should be treated with extreme caution. This unreliability stems from several critical biases that significantly skew the results and prevent accurate generalizations about the larger population. Understanding these biases is crucial for anyone involved in data analysis or interpreting research findings.
The Fundamental Flaws of Voluntary Response Samples
The core problem with voluntary response samples lies in their inherent self-selection bias. This bias arises because the individuals who choose to participate are inherently different from those who do not. Their participation itself reveals something about their attitudes, beliefs, or experiences. For example, a survey about customer satisfaction conducted solely through online feedback forms will likely overrepresent dissatisfied customers, as those with positive experiences are less likely to take the time to submit feedback.
This self-selection bias introduces several significant issues:
1. Overrepresentation of Certain Demographics
Voluntary response samples tend to attract individuals with strong opinions or vested interests in the topic. People who are passionate or deeply affected by the issue at hand are more likely to participate, while those who are neutral or less involved are less inclined. This leads to an overrepresentation of certain demographic groups and viewpoints, resulting in a sample that doesn't accurately reflect the population's true distribution.
For instance, a survey about a controversial political issue conducted via an online forum is likely to be dominated by individuals who hold strong opinions on either side of the debate. The silent majority, those with moderate views or no strong opinions, will be underrepresented or absent entirely. This skewed representation severely limits the generalizability of the findings.
2. Non-Response Bias
A closely related issue is non-response bias. This occurs when a significant portion of the invited individuals choose not to participate, leading to missing data. The reasons for non-participation can be varied, ranging from lack of time or interest to concerns about privacy or the survey's methodology. Crucially, non-respondents often differ systematically from respondents, further biasing the results. They may hold different views or possess different characteristics, leading to a skewed representation of the population.
Ignoring non-response bias can lead to misleading conclusions. For example, a study on health habits using a voluntary response sample might overestimate the prevalence of healthy behaviors because individuals who engage in unhealthy lifestyles are less likely to participate.
3. Lack of Randomization
A hallmark of reliable statistical inference is the use of random sampling. Random sampling ensures that each member of the population has an equal chance of being selected, minimizing the influence of bias and allowing for valid generalizations. Voluntary response samples, by their very nature, lack this crucial element of randomness. Participants are self-selected, introducing a systematic bias that undermines the validity of any conclusions drawn from the data.
Without randomization, it's impossible to assess the extent of the sampling error or to construct valid confidence intervals that accurately reflect the uncertainty associated with estimating population parameters. This makes it difficult, if not impossible, to draw meaningful inferences about the larger population.
Examples of Unreliable Inferences from Voluntary Response Samples
Consider several real-world scenarios to illustrate the pitfalls of relying on voluntary response samples:
1. Online Polls and Surveys
Online polls and surveys are notorious for their susceptibility to bias. They often attract individuals with strong opinions, leading to results that are not representative of the broader population. For example, an online poll asking about public opinion on a political candidate might be heavily skewed towards supporters of that candidate, failing to capture the views of undecided or opposing voters.
2. Call-in Radio Shows and Television Programs
Call-in radio shows and television programs often solicit opinions on various topics. However, the individuals who call in are typically those with strong feelings on the issue, leading to an unrepresentative sample. The views expressed on such platforms rarely reflect the true distribution of opinions within the general population.
3. Mail-in Surveys
While appearing more formal, mail-in surveys can also suffer from significant biases. Response rates are often low, and those who respond may differ systematically from those who do not. For instance, a mail-in survey on health habits might attract individuals who are more health-conscious, leading to an overestimation of the prevalence of healthy behaviors in the population.
4. Feedback Forms
Customer feedback forms, while useful for gathering individual opinions, are not suitable for making general inferences about customer satisfaction. Dissatisfied customers are often more motivated to provide feedback than satisfied ones, leading to a biased perception of overall customer sentiment.
Mitigating the Risks of Voluntary Response Samples
While voluntary response samples are generally unreliable, some strategies can help mitigate their limitations. However, it's crucial to understand that these strategies do not eliminate the underlying biases, but rather attempt to reduce their impact:
-
Triangulation: Combining data from voluntary response samples with data from other sources, such as representative surveys, can provide a more complete picture. By comparing results across multiple data sources, researchers can identify potential biases and gain a more nuanced understanding of the issue.
-
Qualitative Analysis: While not providing statistically significant inferences, qualitative analysis of voluntary response data can offer insights into underlying attitudes and opinions. Careful analysis of the text or narrative responses can reveal valuable qualitative information that complements quantitative findings from other data sources.
-
Acknowledging Limitations: Transparency is key. When reporting results from voluntary response samples, it's crucial to clearly state the limitations of the data and acknowledge the potential biases. This helps readers interpret the findings with appropriate caution and avoids making unwarranted generalizations.
Conclusion: Emphasizing the Importance of Robust Sampling Methods
Inferences based on voluntary response samples are inherently unreliable due to self-selection bias, non-response bias, and the lack of randomization. These biases lead to skewed results that cannot accurately represent the characteristics of the larger population. While certain mitigation strategies can be employed, they do not eliminate the fundamental flaws of this sampling method. For accurate and reliable statistical inferences, it is crucial to employ robust sampling techniques, such as simple random sampling, stratified sampling, or cluster sampling, which ensure representativeness and minimize bias. Ignoring the limitations of voluntary response samples can lead to misleading conclusions, flawed decision-making, and a misrepresentation of reality. Therefore, always prioritize rigorous sampling methods to ensure the validity and reliability of research findings. Only through scientifically sound data collection can we make informed decisions and build a more evidence-based understanding of the world around us. The use of voluntary response samples should be treated with extreme caution and only used for exploratory or qualitative purposes, never as a basis for making generalizations about a population.
Latest Posts
Latest Posts
-
What Is The Easiest And Fastest Nail Art Medium
Apr 04, 2025
-
Herniation Of The Urethra Is Known As
Apr 04, 2025
-
Factor Analysis Has Been Used To Identify The Most Basic
Apr 04, 2025
-
The Party That Raised The Most Money Overall Is
Apr 04, 2025
-
A Weakness Or Slight Muscular Paralysis Is Known As
Apr 04, 2025
Related Post
Thank you for visiting our website which covers about Inferences Based On Voluntary Response Samples Are Generally Not Reliable. . We hope the information provided has been useful to you. Feel free to contact us if you have any questions or need further assistance. See you next time and don't miss to bookmark.