What Can You Surmise From The Labeled Image
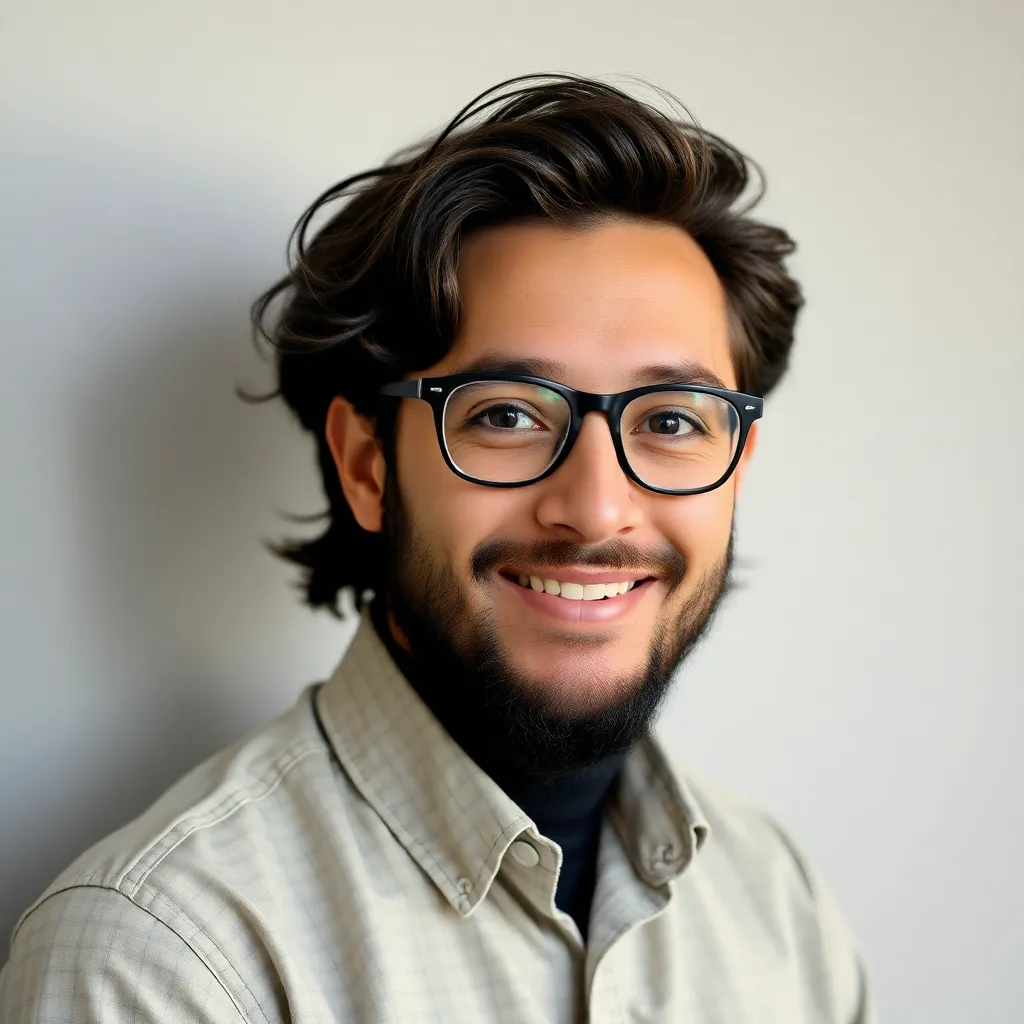
Breaking News Today
May 10, 2025 · 5 min read
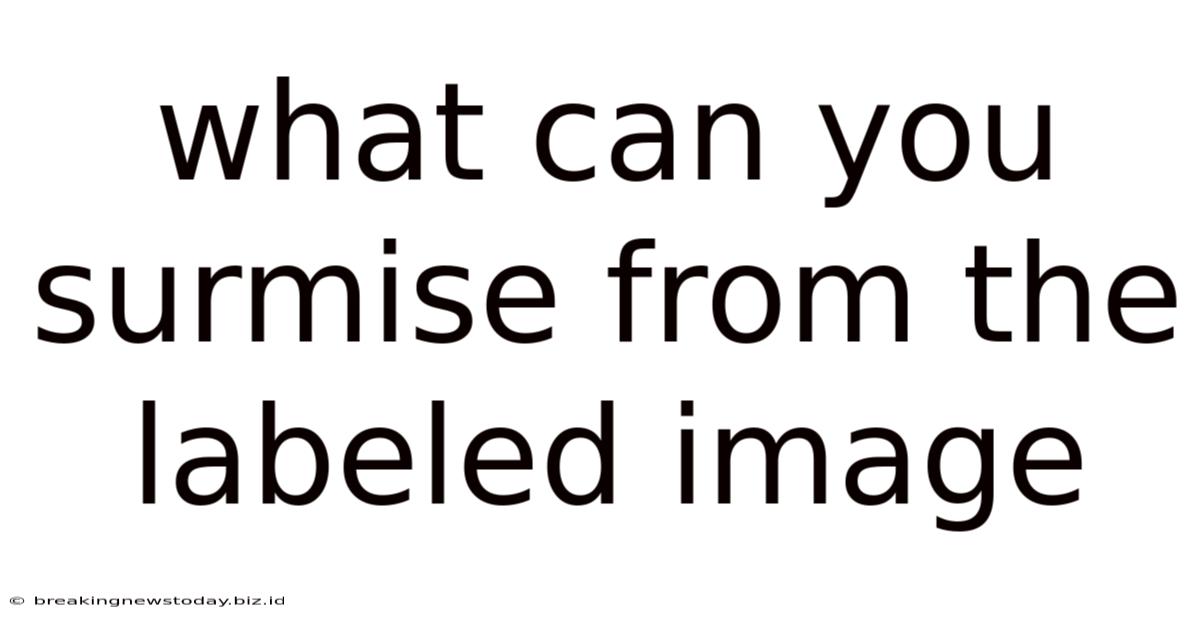
Table of Contents
What Can You Surmise From the Labeled Image? A Deep Dive into Visual Inference
Analyzing images, especially those with labels, goes far beyond simple observation. It involves a complex interplay of visual perception, contextual understanding, and inferential reasoning. This article will explore the various techniques and considerations involved in drawing conclusions from labeled images, highlighting the nuances of interpretation and the limitations of visual data alone.
The Importance of Context in Image Interpretation
Before we dive into specific examples, it's crucial to understand the paramount role of context in interpreting labeled images. A label, in itself, provides only limited information. The true meaning emerges when we consider the broader context:
1. The Source of the Image:
- Scientific research: Images from scientific studies will require a different interpretative lens compared to images from social media. Scientific images are usually accompanied by detailed metadata and experimental protocols, providing crucial context.
- News media: Images from news sources must be scrutinized for potential bias or manipulation. Consider the angle, lighting, and even the captioning.
- Social media: Images on platforms like Instagram or Twitter are often informal and may lack verifiable context. Analyzing the poster's profile and surrounding comments can add crucial layers of understanding.
- Personal archives: Personal photographs may lack explicit labeling but offer rich contextual clues through accompanying memories or stories.
2. The Nature of the Labels:
- Descriptive labels: Simple descriptive labels like "cat" or "tree" offer a basic identification. However, more nuanced labels like "Siamese cat" or "oak tree" provide crucial detail.
- Quantitative labels: Labels providing numerical data (e.g., "temperature: 25°C," "height: 1.8m") significantly enhance our understanding. These labels add precision and allow for quantitative analysis.
- Qualitative labels: Labels describing qualities (e.g., "bright," "sad," "ruined") introduce subjectivity and require careful consideration of the labeler's perspective.
- Ambiguous labels: Poorly defined or ambiguous labels lead to uncertainty and require further investigation to ascertain their precise meaning.
3. The Surrounding Visual Elements:
The labeled object or subject rarely exists in isolation. The surrounding visual elements provide significant contextual cues. Consider:
- Spatial relationships: The position of the labeled object relative to other objects in the image can reveal important information about its function or significance.
- Scale and proportion: The size of the labeled object compared to other objects in the image helps establish its scale and relative importance.
- Color and texture: Color and texture can provide clues about the material composition, age, and condition of the labeled object.
- Lighting and shadow: These elements can affect our perception of depth, shape, and texture.
Developing Inferential Skills: Examples and Analysis
Let's illustrate these principles with some examples. Imagine you have labeled images depicting various scenarios.
Example 1: A labeled image of a "protest" with individuals holding signs saying "Climate Justice Now!"
From this image, we can surmise:
- The primary theme: The image depicts a protest related to climate change.
- The participants' viewpoint: The protesters are advocating for climate justice.
- The potential context: This could be a local or national protest, part of a larger movement, or a spontaneous demonstration.
- Further questions: What is the location? How many participants are present? What specific policies or actions are they demanding?
Example 2: A labeled image of a "microscope slide" showing labeled cells with "cancerous" and "healthy" markings.
This image allows us to infer:
- The subject: The image shows a microscopic view of cells, presumably from a biopsy.
- The diagnosis: Some cells are identified as cancerous, while others are identified as healthy.
- The implications: The image suggests a diagnosis of cancer and the possibility of further medical treatment.
- Further questions: What type of cancer? What is the stage of the cancer? What are the next steps in treatment?
Example 3: A labeled image of a "traffic accident" scene showing a damaged car with "speed limit 30 mph" sign visible.
This labeled image allows for the following inferences:
- The event: A traffic accident has occurred.
- A potential contributing factor: The speed limit sign suggests that speeding might have played a role in the accident (though we cannot confirm this definitively).
- The severity: The extent of car damage hints at the severity of the accident, although further investigation is needed.
- Further questions: Were there injuries? Who was at fault? What were the weather conditions at the time of the accident?
Example 4: A labeled image showing a "historical photograph" of a street scene, labeled with "1920s, New York City."
This image allows us to surmise:
- The time period: The image is from the 1920s.
- The location: The photo was taken in New York City.
- Potential observations about the era: By studying architectural styles, fashion, vehicles, and other details, we can learn about the social and cultural context of the 1920s in New York City.
- Further questions: What specific location within NYC is shown? What is the social class depicted? What activities are visible?
Limitations and Potential Biases in Visual Inference
It is crucial to acknowledge the limitations inherent in drawing inferences solely from labeled images:
- Selection bias: The selection of the image itself can introduce bias. Not all perspectives are captured, leading to an incomplete or skewed representation.
- Labeling bias: Labels themselves can be biased, reflecting the preconceptions and biases of the person creating the labels.
- Incomplete information: Images, even with labels, often provide incomplete information. Critical details may be missing or obscured.
- Misinterpretation: Visual perception is subjective. Different individuals may interpret the same image differently.
- Lack of temporal information: Static images offer a single snapshot in time and do not reveal the events that preceded or followed.
Therefore, it's essential to approach image interpretation with a critical and cautious mindset, acknowledging the potential for biases and limitations. Cross-referencing with other sources of information is vital for a more comprehensive understanding.
Conclusion: From Observation to Informed Inference
Drawing informed conclusions from labeled images involves much more than simple visual observation. It requires a nuanced understanding of context, careful consideration of labeling biases, and a critical appraisal of potential limitations. By mastering the art of visual inference, we can unlock valuable insights from images, converting raw visual data into meaningful knowledge across diverse fields, from scientific research and medical diagnosis to historical analysis and social commentary. However, remember that rigorous analysis, coupled with a healthy dose of skepticism, remains the cornerstone of accurate and reliable interpretation.
Latest Posts
Latest Posts
-
Life Income Joint And Survivor Settlement Option Guarantees
May 10, 2025
-
Which Text Evidence Best Supports The Inference That Jacqueline Overcame
May 10, 2025
-
The Sliding Filament Model Of Contraction Involves
May 10, 2025
-
When Crossing Paths What Is The Give Way Vessels Responsibility
May 10, 2025
-
What Is The Indication For Mouth To Mouth Rescue Breaths Quizlet
May 10, 2025
Related Post
Thank you for visiting our website which covers about What Can You Surmise From The Labeled Image . We hope the information provided has been useful to you. Feel free to contact us if you have any questions or need further assistance. See you next time and don't miss to bookmark.