When Is An Outlier Most Likely To Be Problematic
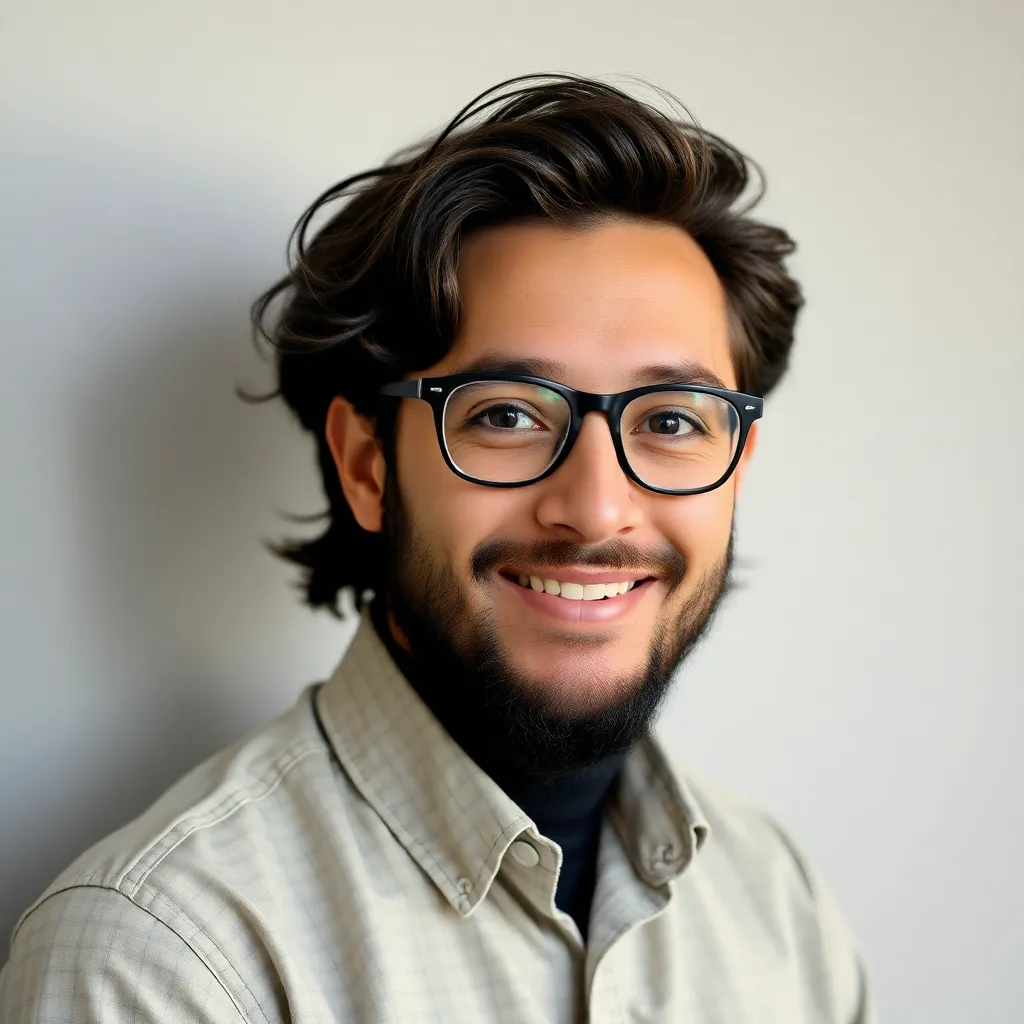
Breaking News Today
Mar 29, 2025 · 6 min read
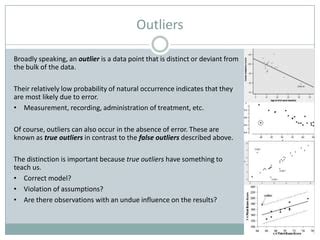
Table of Contents
When is an Outlier Most Likely to Be Problematic?
Outliers, those data points that stray significantly from the rest of the dataset, are a common headache for data analysts and researchers. While sometimes representing genuine anomalies or valuable insights, they often introduce significant problems into statistical analyses, model building, and decision-making. Understanding when an outlier is most likely to be problematic is crucial for effective data handling. This article delves into various scenarios where outliers pose the greatest challenges, exploring their impact on different statistical methods and offering strategies for mitigating their negative effects.
Understanding the Nature of Outliers
Before diving into problematic scenarios, let's clarify what constitutes an outlier. It's not simply a data point that's different; it's one that deviates significantly from the expected pattern or distribution. This deviation can stem from various sources:
-
Data Entry Errors: Simple human error during data collection or input is a frequent cause. A misplaced decimal point or a typo can create a wildly inaccurate data point.
-
Measurement Errors: Faulty equipment, inaccurate measurement techniques, or environmental factors can lead to erroneous measurements that stand out from the rest.
-
Natural Variation: In some cases, outliers might represent genuine, albeit rare, events within the population being studied. These aren't errors but rather extreme values that are part of the natural variability. Distinguishing these from error-induced outliers is a key challenge.
-
Changes in Underlying Processes: An outlier might signal a shift or change in the system generating the data. This could indicate a new phenomenon or a deviation from previously established patterns.
Scenarios Where Outliers Pose Significant Problems
Outliers' impact varies depending on the analytical technique used and the context of the data. Here are some scenarios where outliers are most problematic:
1. Impact on Descriptive Statistics
Descriptive statistics, such as the mean, median, and standard deviation, are highly susceptible to the influence of outliers.
-
Mean: The mean is particularly sensitive. A single extreme value can significantly skew the mean, providing a misleading representation of the central tendency. Consider a dataset of salaries where one individual earns significantly more than others. The mean salary would be inflated, failing to accurately reflect the typical salary. The median, which is less affected by extreme values, becomes a more robust measure of central tendency in such cases.
-
Standard Deviation: Similarly, the standard deviation, a measure of data dispersion, can be inflated by outliers, leading to overestimation of the variability in the data. This can affect confidence intervals and hypothesis testing. Robust measures of dispersion, such as the Interquartile Range (IQR), are less sensitive to outliers.
2. Impact on Inferential Statistics
Outliers can severely distort the results of inferential statistical tests, leading to incorrect conclusions.
-
Hypothesis Testing: Outliers can inflate the variability within the data, leading to a decreased power of statistical tests. This makes it harder to detect significant differences or relationships even when they exist. Conversely, outliers might artificially create a significant result when none truly exists.
-
Regression Analysis: In regression analysis, outliers can significantly influence the regression line, leading to inaccurate predictions and biased coefficient estimates. A single influential outlier can unduly pull the regression line towards itself, misrepresenting the relationship between variables. Robust regression techniques exist to mitigate this problem.
-
Correlation Analysis: Outliers can artificially inflate or deflate the correlation coefficient, distorting the perceived relationship between variables. A seemingly strong correlation might be entirely driven by one or two extreme data points.
3. Impact on Machine Learning Models
Outliers present significant challenges in machine learning.
-
Supervised Learning: In algorithms like linear regression, outliers can significantly affect the model's performance. The model might try to fit the outlier, resulting in a poor fit for the majority of the data. Robust loss functions and regularization techniques can help mitigate this.
-
Clustering: Outliers can distort the results of clustering algorithms. They can create spurious clusters or be misclassified into inappropriate clusters, impacting the overall accuracy of the analysis. Density-based clustering algorithms are less susceptible to outliers.
-
Anomaly Detection: Ironically, outliers are the focus in anomaly detection algorithms. However, even in this context, handling extreme outliers requires careful consideration to avoid false positives or false negatives.
4. Impact on Data Visualization
Outliers can significantly distort the visual representation of the data, making it difficult to discern patterns and trends.
-
Histograms: Outliers can create extreme skewness in histograms, making it difficult to interpret the distribution of the data.
-
Scatter Plots: In scatter plots, outliers can obscure the relationship between variables or create a false impression of a strong relationship.
5. Practical Implications: Real-World Examples
The consequences of ignoring or mishandling outliers can be severe in various real-world applications:
-
Finance: In fraud detection, outliers might represent fraudulent transactions. Ignoring them could lead to significant financial losses.
-
Healthcare: In medical research, outliers might represent patients with rare conditions or adverse reactions to treatments. Understanding these outliers is crucial for improving patient care.
-
Manufacturing: In quality control, outliers might indicate faulty equipment or defective products. Addressing these outliers is crucial for maintaining product quality and preventing costly recalls.
-
Environmental Science: Outliers in environmental data might indicate pollution events or unusual weather patterns. Properly handling these outliers is critical for accurate environmental monitoring and decision-making.
Strategies for Handling Problematic Outliers
The optimal approach to outliers depends on their cause and the context of the analysis. There is no one-size-fits-all solution. Strategies include:
-
Data Cleaning and Error Correction: The first step is to thoroughly investigate the outliers and attempt to identify and correct any data entry or measurement errors.
-
Robust Statistical Methods: Employing robust statistical methods, such as the median instead of the mean and robust regression, helps reduce the influence of outliers on the analysis.
-
Data Transformation: Transformations, such as logarithmic or square root transformations, can sometimes reduce the influence of outliers by compressing the range of the data.
-
Winsorizing or Trimming: Winsorizing replaces extreme values with less extreme values (e.g., replacing the highest value with the 95th percentile). Trimming involves removing a certain percentage of the most extreme values from both tails of the distribution.
-
Outlier Removal: Removing outliers is a last resort and should only be done after careful consideration and justification. It's crucial to document the reasons for removal and to assess the impact of removal on the results.
-
Separate Analysis: Analyze the outliers separately from the main dataset to potentially uncover hidden patterns or insights. These outliers might represent a separate subpopulation with unique characteristics.
-
Non-parametric methods: Consider using non-parametric methods that are less sensitive to the assumptions of data distribution.
Conclusion
Outliers are a pervasive challenge in data analysis, but their problematic nature isn't inherent. The degree to which they impact analyses depends heavily on the context, the analytical methods employed, and the potential causes underlying their existence. By carefully examining the data, understanding the potential sources of outliers, and employing appropriate strategies, data analysts can effectively mitigate the negative effects of these data points and extract valuable insights from their datasets. Remember that dealing with outliers requires a careful balance between rigorous investigation, sound statistical practices, and a deep understanding of the domain knowledge related to the data. Ignoring outliers can lead to erroneous conclusions and flawed decisions, while overly aggressive outlier removal can discard valuable information. A thoughtful and reasoned approach is key to successful data analysis.
Latest Posts
Latest Posts
-
Careers In Business Management And Administration Quizlet
Apr 01, 2025
-
A Records Freeze Includes Which Of The Following
Apr 01, 2025
-
3 To 11 Rule Of Customer Service
Apr 01, 2025
-
Behaviorism Focuses On Making Psychology An Objective Science By
Apr 01, 2025
-
A Lump In The Testes Can Be Caused By Quizlet
Apr 01, 2025
Related Post
Thank you for visiting our website which covers about When Is An Outlier Most Likely To Be Problematic . We hope the information provided has been useful to you. Feel free to contact us if you have any questions or need further assistance. See you next time and don't miss to bookmark.