Which Of The Following Describes The Probability Distribution Below
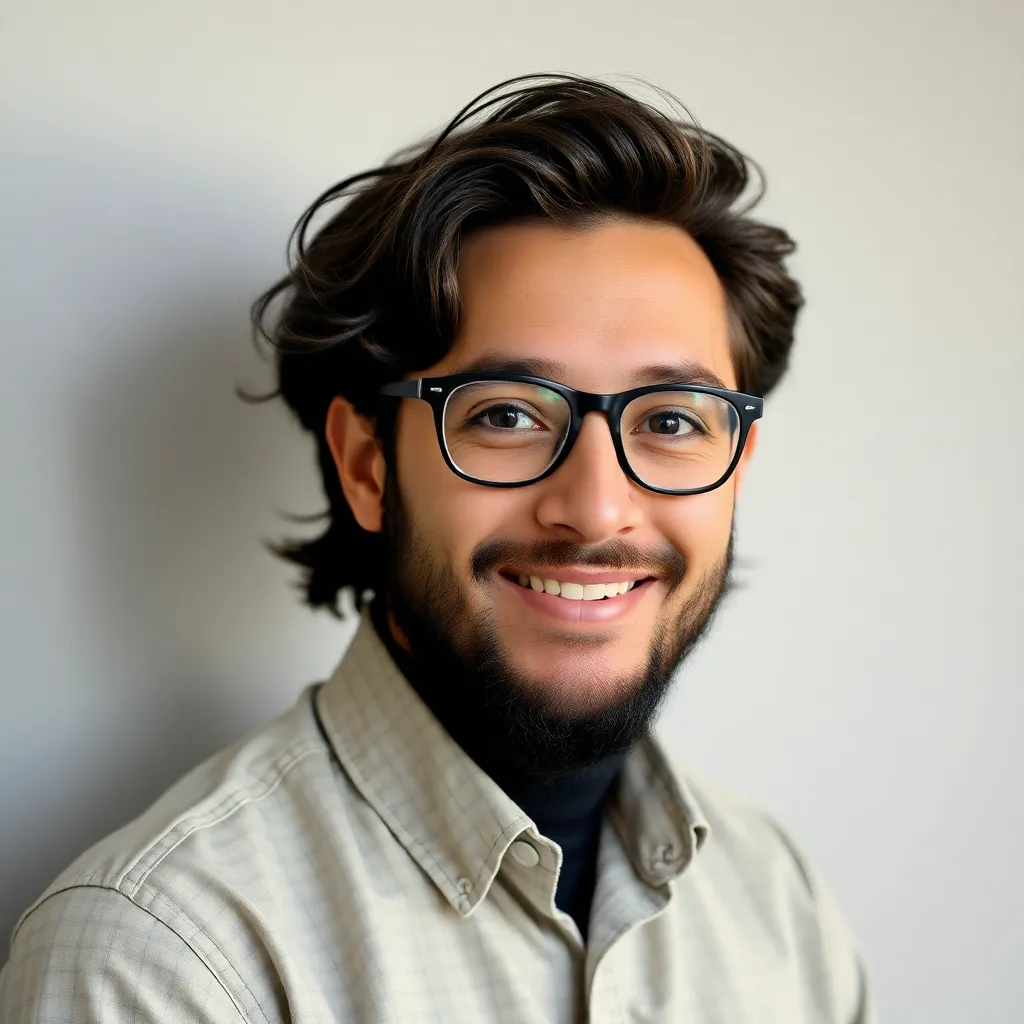
Breaking News Today
May 09, 2025 · 6 min read
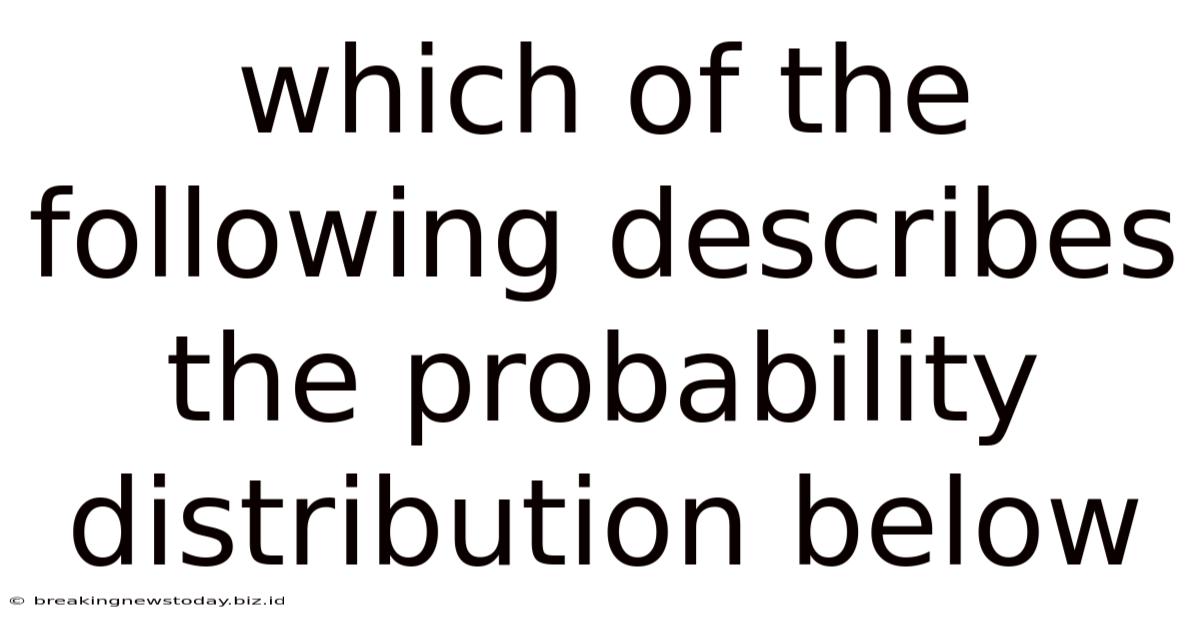
Table of Contents
Decoding Probability Distributions: A Comprehensive Guide
Understanding probability distributions is fundamental to numerous fields, from statistics and machine learning to finance and physics. This article delves deep into identifying and characterizing probability distributions, focusing on how to determine which distribution best describes a given dataset or scenario. We'll cover key characteristics, common distributions, and practical techniques for analysis.
What is a Probability Distribution?
A probability distribution is a mathematical function that provides the probabilities of occurrence of different possible outcomes for a random variable. It essentially maps possible values of a variable to their corresponding probabilities. Understanding the underlying distribution allows us to make predictions, model uncertainty, and draw inferences from data.
Key Characteristics of Probability Distributions
Several key characteristics help identify a specific probability distribution. These include:
-
Type of Variable: Is the variable continuous (can take on any value within a range) or discrete (can only take on specific values)? This significantly narrows down the possibilities.
-
Shape: The visual representation of the distribution, often a histogram or density curve, reveals crucial information. Is it symmetric, skewed (positively or negatively), unimodal (one peak), bimodal (two peaks), or multimodal (multiple peaks)?
-
Central Tendency: This describes the "center" of the distribution. Common measures include the mean (average), median (middle value), and mode (most frequent value). The relationship between these measures offers insights. For instance, a large difference between the mean and median suggests skewness.
-
Spread/Dispersion: This quantifies the variability or spread of the data. Common measures include the range (difference between the maximum and minimum values), variance (average squared deviation from the mean), and standard deviation (square root of the variance).
-
Support: This refers to the range of values the random variable can take. For instance, a uniform distribution has a support defined by its minimum and maximum values, while an exponential distribution has support from zero to infinity.
Common Probability Distributions
Many probability distributions exist, each suited to specific scenarios. Some of the most frequently encountered include:
1. Normal (Gaussian) Distribution:
-
Characteristics: Symmetrical, bell-shaped curve with a single peak at the mean. The mean, median, and mode are equal. Completely defined by its mean (μ) and standard deviation (σ).
-
Applications: Widely used in various fields due to the Central Limit Theorem, which states that the average of a large number of independent and identically distributed random variables tends towards a normal distribution, regardless of the original distribution. Used extensively in statistical inference and hypothesis testing.
2. Binomial Distribution:
-
Characteristics: Discrete distribution describing the probability of getting a certain number of successes in a fixed number of independent Bernoulli trials (each trial has only two possible outcomes: success or failure). Defined by the number of trials (n) and the probability of success in each trial (p).
-
Applications: Modeling the number of heads in a series of coin flips, the number of defective items in a batch, or the number of customers who make a purchase.
3. Poisson Distribution:
-
Characteristics: Discrete distribution describing the probability of a given number of events occurring in a fixed interval of time or space, given a known average rate of occurrence (λ).
-
Applications: Modeling the number of cars passing a point on a highway in an hour, the number of calls received by a call center in a day, or the number of defects in a manufactured product.
4. Uniform Distribution:
-
Characteristics: Describes a random variable with a constant probability density over a specified range. All values within the range have equal probability.
-
Applications: Simulating random numbers, representing uncertainty when limited information is available, and in certain Monte Carlo simulations.
5. Exponential Distribution:
-
Characteristics: Continuous distribution describing the time between events in a Poisson process. Characterized by a single parameter, λ, which represents the rate of events.
-
Applications: Modeling the lifespan of electronic components, the time until a machine fails, or the duration of a phone call.
6. Beta Distribution:
-
Characteristics: Continuous distribution defined on the interval [0, 1], often used to model probabilities or proportions. Has two shape parameters, α and β, which control its shape.
-
Applications: Bayesian statistics, modeling proportions, and representing uncertainty about a probability.
7. Gamma Distribution:
-
Characteristics: Continuous distribution with a wide range of shapes, depending on its shape (k) and scale (θ) parameters. It's often used to model waiting times or positive continuous variables.
-
Applications: Modeling the time until a certain number of events occur, representing the amount of rainfall in a region, or analyzing survival times.
8. Weibull Distribution:
-
Characteristics: Continuous distribution commonly used to model time-to-failure data. It has two parameters, a scale parameter and a shape parameter. The shape parameter dictates the distribution's shape and the scale parameter affects its scale.
-
Applications: Reliability engineering, analyzing the lifespan of equipment, modeling failure rates, and analyzing survival data.
How to Determine the Appropriate Distribution
Identifying the correct probability distribution for a given dataset involves a combination of visual inspection, statistical analysis, and domain knowledge. Here's a structured approach:
-
Visual Inspection: Create a histogram or density plot of the data. Observe the shape – is it symmetrical, skewed, unimodal, or multimodal? This initial visualization provides valuable clues.
-
Descriptive Statistics: Calculate the mean, median, mode, variance, and standard deviation. Compare these measures to identify potential distributions. For example, a large difference between the mean and median suggests skewness, hinting at distributions like the exponential or Weibull.
-
Quantile-Quantile (Q-Q) Plots: These plots compare the quantiles of the data to the quantiles of a theoretical distribution. If the points fall approximately along a straight line, it suggests a good fit.
-
Goodness-of-Fit Tests: Statistical tests, like the Kolmogorov-Smirnov test or the Chi-squared test, can assess how well the data conforms to a specific distribution. These tests provide a quantitative measure of the fit.
-
Domain Knowledge: Consider the context of the data. Are there physical or theoretical reasons to expect a particular distribution? This knowledge can significantly guide the selection process. For example, if modeling time until failure, the exponential or Weibull might be appropriate.
Advanced Techniques
For more complex scenarios, advanced techniques might be necessary:
-
Maximum Likelihood Estimation (MLE): This method estimates the parameters of a distribution that maximize the likelihood of observing the given data.
-
Bayesian Inference: This approach incorporates prior knowledge about the distribution to update beliefs based on the observed data.
-
Mixture Models: These models combine multiple distributions to represent data with heterogeneous characteristics.
Conclusion
Choosing the right probability distribution is crucial for accurate modeling and analysis. By carefully considering the characteristics of your data, employing visual inspection, statistical tests, and domain knowledge, you can effectively identify the best-fitting distribution and gain valuable insights from your data. Remember that no single method is foolproof; a combination of approaches is often needed for robust results. The process is iterative, and you may need to explore multiple distributions before finding the one that best describes your data and supports your analysis effectively.
Latest Posts
Latest Posts
-
Category A Samples Can Be Mixed With Category B
May 09, 2025
-
Blackout Or Get Drunk Trying Card Game
May 09, 2025
-
When Film Emulsion Interacts With X Rays The Result Is
May 09, 2025
-
Indexing Works With Both Strings And Lists
May 09, 2025
-
Which Of The Following Compete For Space On Intertidal Rocks
May 09, 2025
Related Post
Thank you for visiting our website which covers about Which Of The Following Describes The Probability Distribution Below . We hope the information provided has been useful to you. Feel free to contact us if you have any questions or need further assistance. See you next time and don't miss to bookmark.