Which Of The Following Research Designs Will Allow Cause-and-effect Conclusions
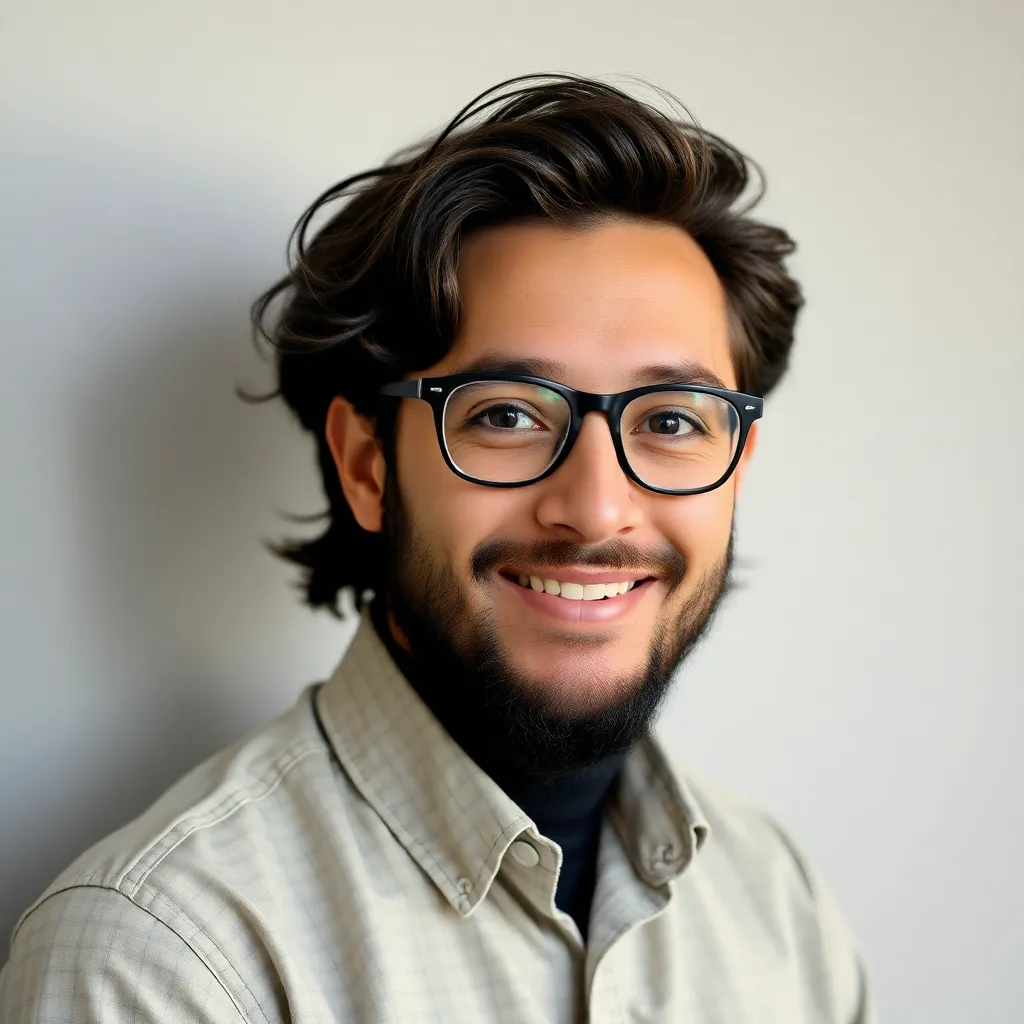
Breaking News Today
Mar 31, 2025 · 6 min read
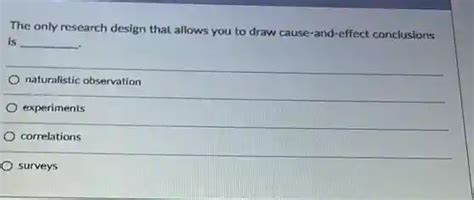
Table of Contents
Which Research Designs Allow Cause-and-Effect Conclusions?
Determining causality—understanding that one variable directly influences another—is a cornerstone of impactful research. While correlation shows a relationship between variables, it doesn't prove causation. To confidently conclude cause-and-effect, specific research designs are necessary. This article delves into the research designs that best allow for drawing such conclusions, highlighting their strengths and limitations.
Understanding Causality: The Three Pillars
Before exploring research designs, it's crucial to understand the three fundamental criteria for establishing causality:
-
Temporal Precedence: The cause (independent variable) must precede the effect (dependent variable) in time. The cause must happen before the effect.
-
Covariation of Cause and Effect: A change in the independent variable must be associated with a change in the dependent variable. There must be a demonstrable relationship between the two.
-
No Plausible Alternative Explanations: This is arguably the most challenging criterion. Researchers must eliminate other potential factors (confounding variables) that could explain the observed relationship between the independent and dependent variables.
Research Designs that Support Causal Inference
Several research designs are specifically designed to address these criteria and allow for stronger causal inferences. These designs are characterized by their control over extraneous variables and their ability to manipulate the independent variable.
1. True Experiments
True experiments, often considered the gold standard for establishing causality, involve the random assignment of participants to different conditions (experimental and control groups). This random assignment is crucial because it minimizes the influence of confounding variables, ensuring that the groups are comparable at the outset.
Key Features of True Experiments:
- Manipulation of the Independent Variable: The researcher directly manipulates the independent variable to observe its effect on the dependent variable.
- Random Assignment: Participants are randomly assigned to either the experimental group (receiving the treatment or manipulation) or the control group (not receiving the treatment).
- Control Group: A control group provides a baseline for comparison, allowing researchers to isolate the effect of the independent variable.
Types of True Experiments:
- Pre-test-Post-test Control Group Design: Measures the dependent variable before and after the manipulation in both the experimental and control groups. This design allows for assessing the change in the dependent variable due to the independent variable.
- Post-test-Only Control Group Design: Measures the dependent variable only after the manipulation. This design is useful when pre-testing might influence the results.
- Solomon Four-Group Design: Combines both pre-test-post-test and post-test-only designs, helping to identify the potential impact of pre-testing on the results.
Strengths of True Experiments:
- Strong internal validity: The ability to confidently attribute changes in the dependent variable to the manipulation of the independent variable.
- Allows for causal inferences: The rigorous control minimizes confounding variables, strengthening the ability to conclude cause-and-effect.
Limitations of True Experiments:
- Artificiality: The controlled environment of a true experiment might not accurately reflect real-world situations, potentially limiting external validity (generalizability).
- Ethical Concerns: Random assignment might not always be ethical, particularly when dealing with potentially harmful or beneficial treatments.
- Practical Limitations: Random assignment can be difficult or impossible in some situations.
2. Quasi-Experiments
Quasi-experiments share similarities with true experiments but lack the crucial element of random assignment. Participants are assigned to groups based on pre-existing characteristics (e.g., gender, age, school). While they don't offer the same level of control over confounding variables as true experiments, they provide valuable insights when random assignment is impossible or impractical.
Key Features of Quasi-Experiments:
- Manipulation of the Independent Variable: Similar to true experiments, the independent variable is manipulated.
- Non-random Assignment: Participants are assigned to groups based on pre-existing characteristics.
- Comparison Groups: Quasi-experiments use comparison groups instead of control groups, although the groups might not be perfectly comparable.
Types of Quasi-Experiments:
- Non-equivalent Control Group Design: Compares an experimental group with a non-equivalent control group.
- Interrupted Time Series Design: Measures the dependent variable repeatedly before and after an intervention or event.
- Regression Discontinuity Design: Assigns participants to groups based on a cut-off score, examining the effect of crossing the threshold.
Strengths of Quasi-Experiments:
- Practicality: They are feasible in situations where random assignment is impossible or unethical.
- Real-world applicability: They often take place in more natural settings, enhancing external validity.
Limitations of Quasi-Experiments:
- Weaker internal validity: The lack of random assignment increases the risk of confounding variables influencing the results. Causal inferences are weaker compared to true experiments.
- Difficulty in ruling out alternative explanations: More challenging to establish causality due to potential confounding factors.
3. Observational Studies
Observational studies are used when manipulating the independent variable is unethical, impractical, or impossible. Researchers observe and measure variables without interfering. While they cannot establish causality definitively, they can suggest potential causal relationships that can be investigated further.
Types of Observational Studies:
- Cohort Studies: Follow a group of individuals over time to observe the development of a particular outcome.
- Case-Control Studies: Compare individuals with a particular outcome (cases) to individuals without the outcome (controls) to identify potential risk factors.
- Cross-Sectional Studies: Collect data from a sample at a single point in time.
Strengths of Observational Studies:
- Ethical considerations: Suitable for studying sensitive topics or when manipulation is impossible.
- Real-world setting: Often conducted in natural settings, increasing external validity.
- Cost-effective: Can be less resource-intensive than experimental designs.
Limitations of Observational Studies:
- Weak causal inferences: Cannot establish causality conclusively due to the lack of manipulation and control over confounding variables.
- Potential for bias: Selection bias and other biases can influence the results.
- Correlation does not equal causation: Observational studies can only show associations, not causal relationships.
Strengthening Causal Inferences in Non-Experimental Designs
While true experiments provide the strongest evidence for causality, researchers can employ strategies to bolster causal inferences in quasi-experiments and observational studies:
- Statistical Control: Using statistical techniques to account for the effects of confounding variables.
- Matching: Creating comparable groups by matching participants on relevant characteristics.
- Instrumental Variables: Using a third variable to indirectly assess the effect of the independent variable on the dependent variable.
- Propensity Score Matching: A statistical method used to create comparable groups in observational studies.
Conclusion
Establishing cause-and-effect relationships is a complex endeavor. While true experiments offer the most robust means of achieving this, quasi-experiments and even observational studies, when carefully designed and analyzed, can contribute valuable insights into potential causal relationships. Researchers must carefully consider the strengths and limitations of each design and employ appropriate strategies to minimize bias and strengthen causal inferences. Understanding the nuances of each research design is critical for interpreting research findings and drawing meaningful conclusions about cause-and-effect relationships. The choice of research design should always align with the research question, available resources, and ethical considerations.
Latest Posts
Latest Posts
-
Exchange Rate And Purchasing Power Quick Check
Apr 02, 2025
-
Provide An Appropriate Article And Noun For Each Picture
Apr 02, 2025
-
Pablo Y Mercedes Al Aeropuerto En Autobus
Apr 02, 2025
-
The Greek Words Demos And Kratis Together Mean
Apr 02, 2025
-
Essentials Of Radiographic Physics And Imaging Chapter 1
Apr 02, 2025
Related Post
Thank you for visiting our website which covers about Which Of The Following Research Designs Will Allow Cause-and-effect Conclusions . We hope the information provided has been useful to you. Feel free to contact us if you have any questions or need further assistance. See you next time and don't miss to bookmark.