Which Of The Following Is Not True About Machine Learning
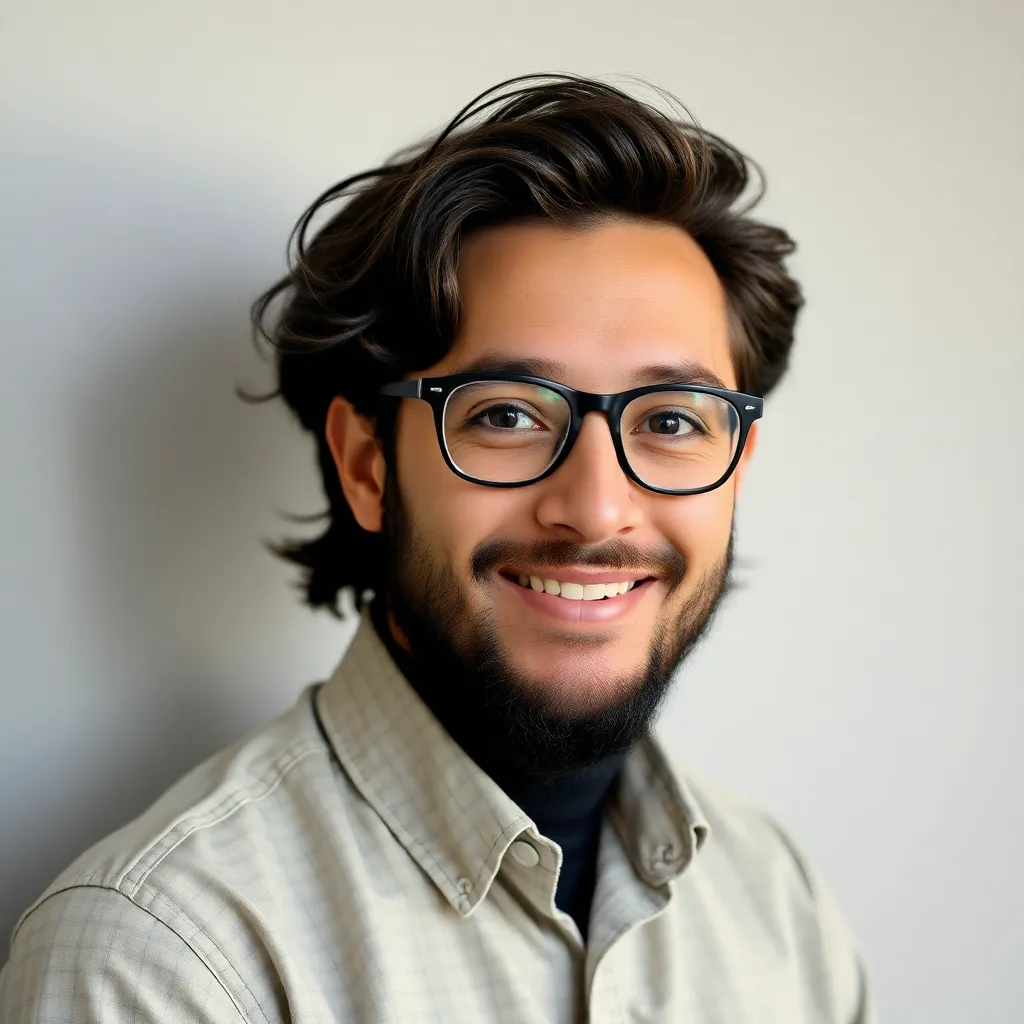
Breaking News Today
Mar 28, 2025 · 6 min read
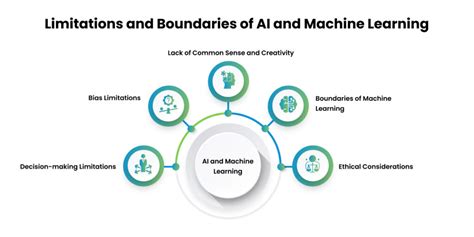
Table of Contents
Which of the Following is NOT True About Machine Learning? Debunking Common Myths
Machine learning (ML) is rapidly transforming our world, powering everything from self-driving cars to personalized recommendations. Its potential is vast, but understanding what machine learning can't do is just as important as understanding what it can. This article dives deep into common misconceptions surrounding machine learning, debunking myths and clarifying the realities of this powerful technology.
Myth 1: Machine Learning is Magic; It Can Solve Any Problem
This is perhaps the biggest misconception. While machine learning offers incredible capabilities, it's not a silver bullet. It's a powerful tool, but like any tool, its effectiveness depends heavily on the context, the data used, and the expertise of the people using it.
Reality Check: The Limitations of Data
ML algorithms thrive on data. Garbage in, garbage out is a fundamental principle in machine learning. If the data used to train a model is biased, incomplete, or noisy, the resulting model will likely be unreliable and inaccurate. For example, a facial recognition system trained primarily on images of light-skinned individuals will likely perform poorly on images of people with darker skin tones. This highlights the crucial need for diverse and representative datasets.
Furthermore, many real-world problems are simply too complex to be solved solely by machine learning. Factors like human intuition, creativity, and ethical considerations often play a crucial role. ML can be a valuable component of a solution, but it's rarely the complete answer.
Myth 2: Machine Learning Requires Massive Amounts of Data
While large datasets are often beneficial for training sophisticated ML models, it's not always a necessity. Many successful ML applications use relatively small datasets, particularly those utilizing techniques like transfer learning or techniques suited for low-data regimes.
Reality Check: The Power of Transfer Learning and Data Augmentation
Transfer learning, for example, leverages pre-trained models (trained on massive datasets) and adapts them to new tasks with smaller datasets. This significantly reduces the amount of data required for training and allows for faster development. Additionally, data augmentation techniques artificially increase the size of a dataset by creating modified versions of existing data points. This is particularly useful in image recognition, where variations in lighting, angles, and scale can be artificially introduced.
Therefore, while larger datasets often lead to better performance, it's crucial to understand that the amount of data needed is highly dependent on the specific task, the algorithm used, and the availability of pre-trained models.
Myth 3: Machine Learning Models are Always Accurate and Reliable
This is a dangerous misconception. ML models are essentially sophisticated statistical models, and as such, they are subject to error and uncertainty. The accuracy of a model depends heavily on the quality of the data used to train it, the chosen algorithm, and the specific task at hand.
Reality Check: Understanding Error and Uncertainty
Even the most sophisticated ML models can make mistakes. These errors can stem from various sources, including noisy data, model limitations, and unforeseen circumstances. Therefore, it's crucial to evaluate the performance of an ML model thoroughly using appropriate metrics and techniques like cross-validation. Furthermore, understanding the limitations of a model and its potential sources of error is essential for responsible deployment. Blind faith in the accuracy of an ML model can lead to disastrous consequences.
Myth 4: Machine Learning is Fully Automated and Requires No Human Intervention
While ML algorithms automate certain aspects of the process, human expertise is crucial at every stage, from data collection and preprocessing to model evaluation and deployment.
Reality Check: The Human in the Loop
The development and deployment of ML systems require significant human intervention. Humans are responsible for:
- Data Collection and Preprocessing: This involves gathering relevant data, cleaning it, and preparing it for use in training. This is often the most time-consuming and crucial step.
- Feature Engineering: Selecting and transforming relevant features from the raw data is a critical step that heavily influences model performance. This requires deep domain expertise.
- Model Selection and Training: Choosing the appropriate algorithm and tuning its hyperparameters requires expertise and experimentation.
- Model Evaluation and Deployment: Assessing the performance of a model and deploying it into a production environment requires careful consideration and monitoring.
- Ongoing Monitoring and Maintenance: ML models need continuous monitoring and updates to maintain accuracy and reliability. Data drift and concept drift are ever-present challenges.
Ignoring the human element in the development and deployment of ML systems is a recipe for failure.
Myth 5: Machine Learning Models are Easy to Understand and Interpret
Many sophisticated ML models, particularly deep learning models, are often referred to as "black boxes." This means that it can be difficult to understand exactly how they arrive at their predictions.
Reality Check: Explainability and Interpretability
The lack of interpretability can be a significant limitation, particularly in applications where understanding the decision-making process is crucial (e.g., medical diagnosis, loan applications). While some ML models are inherently more interpretable than others (e.g., decision trees), the quest for more explainable AI (XAI) is a rapidly growing area of research. Techniques like SHAP (SHapley Additive exPlanations) and LIME (Local Interpretable Model-agnostic Explanations) are being developed to improve the interpretability of complex models. However, achieving full transparency in all cases remains a challenge.
Myth 6: Machine Learning Will Replace Human Jobs Entirely
This is a common fear, but the reality is more nuanced. While ML can automate certain tasks, it's more likely to augment human capabilities rather than entirely replace human jobs.
Reality Check: Augmentation, Not Replacement
ML is likely to transform the job market, creating new roles while rendering others obsolete. However, many jobs will require humans to work alongside ML systems, utilizing their skills to oversee, interpret, and refine the outputs of these systems. The focus should be on reskilling and upskilling the workforce to adapt to the changing demands of the job market.
Myth 7: Machine Learning is Only for Tech Giants
While large tech companies have the resources to develop and deploy sophisticated ML systems, the field is becoming increasingly accessible to individuals and smaller organizations.
Reality Check: The Democratization of Machine Learning
Cloud-based machine learning platforms, open-source libraries, and readily available datasets have significantly lowered the barrier to entry. Many powerful ML tools are now accessible through user-friendly interfaces, empowering individuals and smaller organizations to leverage the power of ML without requiring extensive expertise in computer science or mathematics.
Conclusion: Navigating the Realities of Machine Learning
Machine learning is a powerful technology with the potential to revolutionize many aspects of our lives. However, it's essential to understand its limitations and avoid common misconceptions. By understanding what machine learning can and cannot do, we can harness its power responsibly and ethically, maximizing its benefits while mitigating its risks. The future of machine learning lies in a collaborative approach, combining the strengths of humans and machines to solve complex problems and build a better future. Remember, machine learning is a tool, and its effectiveness depends entirely on how we choose to use it.
Latest Posts
Latest Posts
-
Commercial Banks Create Money When They Quizlet
Mar 30, 2025
-
Basics And The Time Value Of Money Quizlet
Mar 30, 2025
-
Careers In Government And Public Administration Quizlet
Mar 30, 2025
-
The Actions Of The Internal Obliques Include
Mar 30, 2025
-
The Division Of The Cytoplasm Is Called
Mar 30, 2025
Related Post
Thank you for visiting our website which covers about Which Of The Following Is Not True About Machine Learning . We hope the information provided has been useful to you. Feel free to contact us if you have any questions or need further assistance. See you next time and don't miss to bookmark.